RESULTS: REcommender Systems for engaging Users with healthy Living habiTS

The DSRS research group is organising an exciting interdisciplinary workshop, as part of the 2019 Data Week celebrated next May at Bristol’s Jean Golding Institute. See below for event information and our three confirmed speakers’ profile.
Registration: register for this event via Eventbrite (PLACES LIMITED)
Event enquiries: Please contact Iván Palomares Carrascosa, workshop chair, for any event-related enquiries: i.palomares (at) bristol.ac.uk
Overview
This workshop is aimed at researchers, students, academics and practitioners across the Turing network of UK universities and beyond, as well as members of related industry sectors.
There will be a discussion forum on the challenges and opportunities of personalisation approaches, data-driven Decision Support and Recommender Systems (RecSys) in the areas of fitness, wellbeing and promoting healthy living. We seek to bring together experts across the disciplines of Data Science, Decision Support and AI, Medical Sciences, Data Ethics, Psychology and Behavioural Sciences, Nutrition and Sports/Physical Activity.
Event focus
- Connecting experts across disciplines (health, nutrition and sport, etc.) with scientists in Data Science/AI and industry, to put forward potential solutions to unaddressed challenges involving personalisation and/or decision support
- Data ethics implications and user data protection regulations in personalized healthcare and wellbeing.
Participants with ongoing research activity on decision support approaches or RecSys for the health and wellbeing domains are encouraged to bring their laptops to showcase or discuss their results with experts in the field.
Daniele Quercia, King’s College London & Nokia Bell Labs.
Healthy Cities: Tracking Population Health from Grocery Bags and Smart Watches
Bio: Daniele Quercia is with the Department Head of Social Dynamics at Nokia Bell Labs Cambridge (UK) and Professor of Urban Informatics at the Center for Urban Science and Progress (CUSP) at King’s College London. He has been named one of Fortune magazine’s 2014 Data All-Stars, and spoke about “happy maps” at TED. His research has been focusing in the area of urban informatics and received best paper awards from Ubicomp 2014 and from ICWSM 2015, and an honourable mention from ICWSM 2013. He was Research Scientist at Yahoo Labs, a Horizon senior researcher at the University of Cambridge, and Postdoctoral Associate at the department of Urban Studies and Planning at MIT. He received his PhD from UC London. His thesis was sponsored by Microsoft Research and was nominated for BCS Best British PhD dissertation in Computer Science.
Abstract: We will see how to aggregate both readings from consumer wearable devices and records of food purchases to track people’s well-being at scale. From 11,600 Nokia Health wearables, we collected readings of steps, sleep, and heart rate in the entire cities of London and San Francisco over the course of 1 year. Christmas and New Year’s eve were associated only with short-lived and minor disruptions, while both Brexit and Trump’s election greatly impacted people’s sleep and even heart rates. Then, for another entire year in London, we studied the association between food purchases in grocery stores, as measured by the digital traces of customer loyalty cards, and consumption of medicines. Our results show that analytics of digital records of grocery purchases can be used as a cheap and scalable tool for health surveillance: the distribution of the food nutrients is far more predictive of food-related illnesses (e.g. diabetes) than socio-economic conditions.
Balancing the Healthy with the Tasty: Recommending Nutritious Food that People Will Actually Want to Eat
Bio: Morgan Harvey is a Senior Lecturer of information science in the Department of Computer and Information Sciences at Northumbria University, Newcastle. He has been conducting research in the fields of information behaviour and retrieval and recommender systems for over a decade and has published over 50 peer-reviewed conference papers and journal articles, including in ACM SIGIR, ACM CIKM and ACM RecSys. Morgan received his PhD from the University of Strathclyde under the supervision of Prof. Ian Ruthven and has worked as a researcher at the University of Erlangen-Nuremberg in Germany and the University of Lugano in Switzerland.
Abstract: Poor dietary habits are a major cause of today’s world health problems, especially in the developed world. Evidence shows that such issues can be prevented and sometimes even reversed through good nutrition, however, people are often very poor at judging the healthiness of their own diet and need support to implement positive changes. Computer and information technology, and particularly recommender systems, have been suggested as potential solutions to some of these problems but much of the necessary work is yet to be done. This talk will discuss existing research on the problem of recipe recommendation, the difficulty of finding a balance between healthy and enjoyable choices and early work investigating how people can be “nudged” into making healthier food choices.
Multidimensional physical activity: using the right data from wearable fitness trackers and providing the right feedback
Bio: Max is a lecturer in behavioural science within the Department for Health at the University of Bath. He has keen research interests in the factors that influence and facilitate health-enhancing physical activity behaviour, the application of digital health technologies for measurement and intervention and healthy ageing. In recent years Max has worked on several large lifestyle-based intervention trials funded by the MRC and NIHR. Specifically, he has helped develop and evaluate interdisciplinary projects aimed at reducing risk of future chronic disease using wearable monitoring technology to provide feedback on multidimensional physical activity; combatting physical frailty in older age through a community-based physical activity programme; and reducing the decline in cognitive function in older adults with mild-cognitive impairment using a digital web-based behaviour change intervention.
Abstract: The benefits of regular physical activity behaviour on physical function and health are well documented and it is apparent that there are multiple dimensions to physical activity are important. The accurate measurement of physical activity is key to understanding the relationship between behaviour and health, providing credible feedback to participants and judging the success of behaviour change interventions aimed at increasing levels. Accelerometers are popularised as being the most objective and accurate format of assessment. This talk will draw on research data to discuss the strengths and limitations to their current use, such as their (in)appropriateness for comparisons with public health guidelines and the interpretation of what counts as physical activity, and propose solutions to these issues that should guide future applications of these measurement devices.
Event co-sponsored by: Jean Golding Institute for Data-Intensive Research, University of Bristol, and Bristol’s Intelligent Systems Lab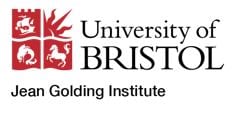